Visual Results
JarvisIR demonstrates superior image restoration capabilities across various real-world degradation scenarios. Explore our results below.
Abstract
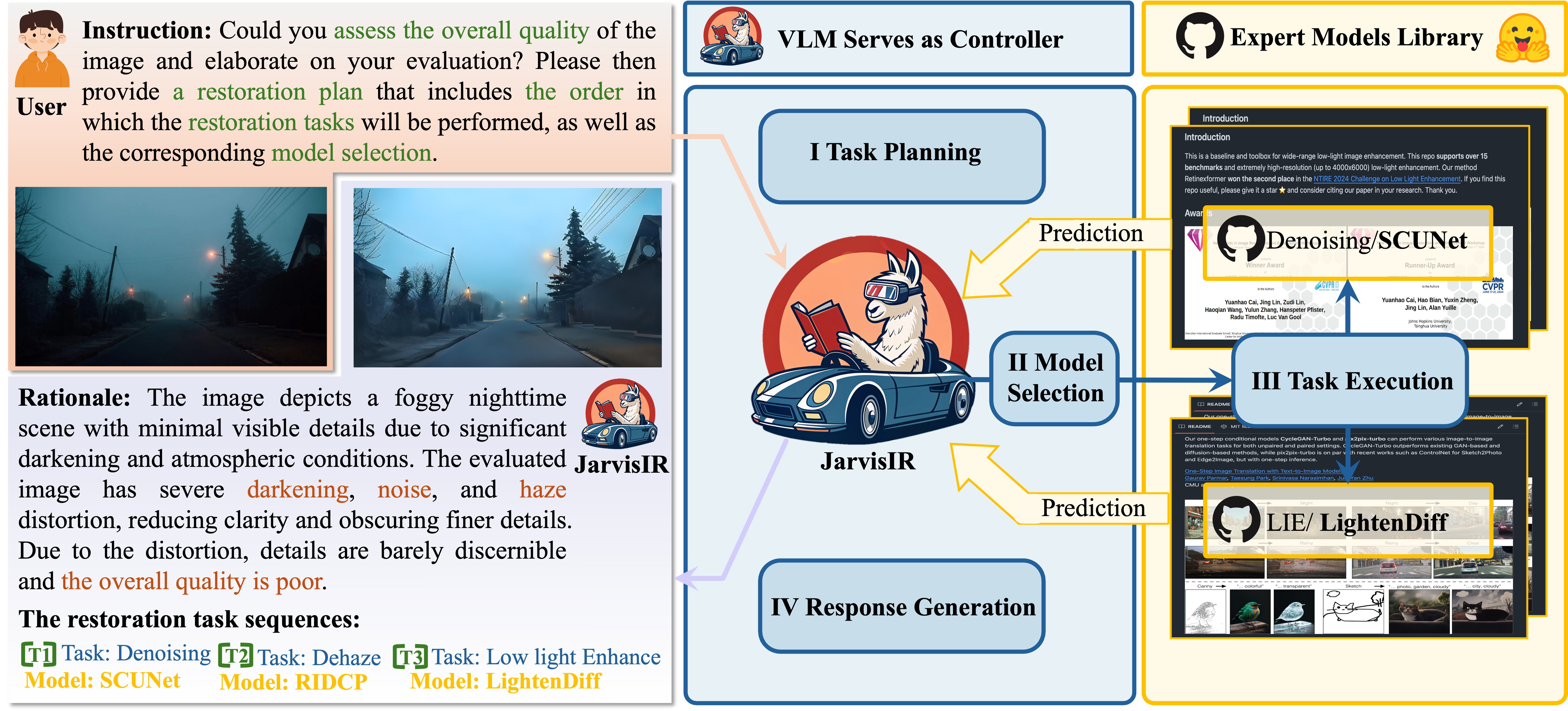
Figure 1: The workflow of JarvisIR. To address real-world coupled weather degradation, we develop JarvisIR, a VLM-powered intelligent system that dynamically schedules expert models for restoration. Initially, JarvisIR assesses the degradation of the input images and parses user instructions to formulate a task plan, selecting the appropriate expert models for each subtask. The selected experts perform their designated tasks and return the results to JarvisIR, which integrates the outcomes and provides the final answer to the user. The design of the figure is inspired by HuggingGPT.
Vision-centric perception systems struggle with unpredictable and coupled weather degradations in the wild. Current solutions are often limited, as they either depend on specific degradation priors or suffer from significant domain gaps. To enable robust and autonomous operation in real-world conditions, we propose JarvisIR, a VLM-powered agent that leverages the VLM as a controller to manage multiple expert restoration models.
To further enhance system robustness, reduce hallucinations, and improve generalizability in real-world adverse weather, JarvisIR employs a novel two-stage framework consisting of supervised fine-tuning and human feedback alignment. Specifically, to address the lack of paired data in real-world scenarios, the human feedback alignment enables the VLM to be fine-tuned effectively on large-scale real-world data in an unsupervised manner.
To support the training and evaluation of JarvisIR, we introduce CleanBench, a comprehensive dataset consisting of high-quality and large-scale instruction-responses pairs, including 150K synthetic entries and 80K real entries. Extensive experiments demonstrate that JarvisIR exhibits superior decision-making and restoration capabilities. Compared with existing methods, it achieves a 50% improvement in the average of all perception metrics on CleanBench-Real.
Method
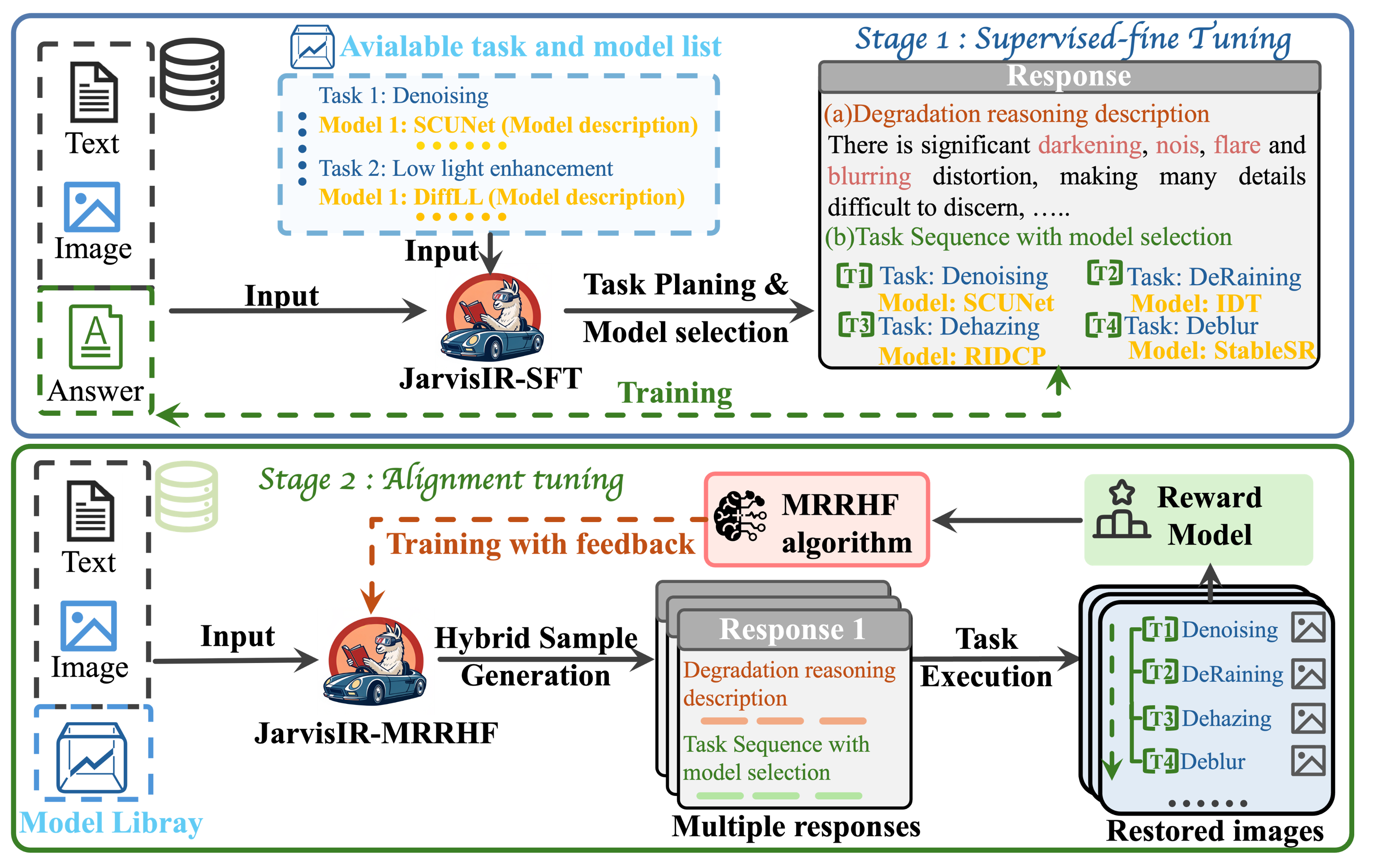
Figure 2: Two-stage training framework of JarvisIR. In the first stage, JarvisIR undergoes supervised fine-tuning on synthetic data from CleanBench to enable it to follow user instructions and recognize image degradation. In the second stage, we further finetune JarvisIR on CleanBench-Real using the MRRHF algorithm to improve system robustness, reduce hallucinations, and enhance generalizability under real-world adverse weather conditions.
Key Contributions
- We propose JarvisIR, a VLM-powered agent that leverages vision-language models as controllers to manage multiple expert restoration models for robust image restoration in autonomous driving.
- We develop a novel two-stage framework with supervised fine-tuning and human feedback alignment to enhance system robustness in real-world adverse weather conditions.
- We introduce CleanBench, a comprehensive dataset with 150K synthetic and 80K real instruction-response pairs for training and evaluation.
- Extensive experiments demonstrate that JarvisIR achieves a 50% improvement in perception metrics compared to existing methods.
CleanBench Dataset
To support the training and evaluation of JarvisIR, we introduce CleanBench, the first high-quality instruction-following dataset specifically curated for developing intelligent restoration systems, containing 150K synthetic and 80K real instruction-response pairs.
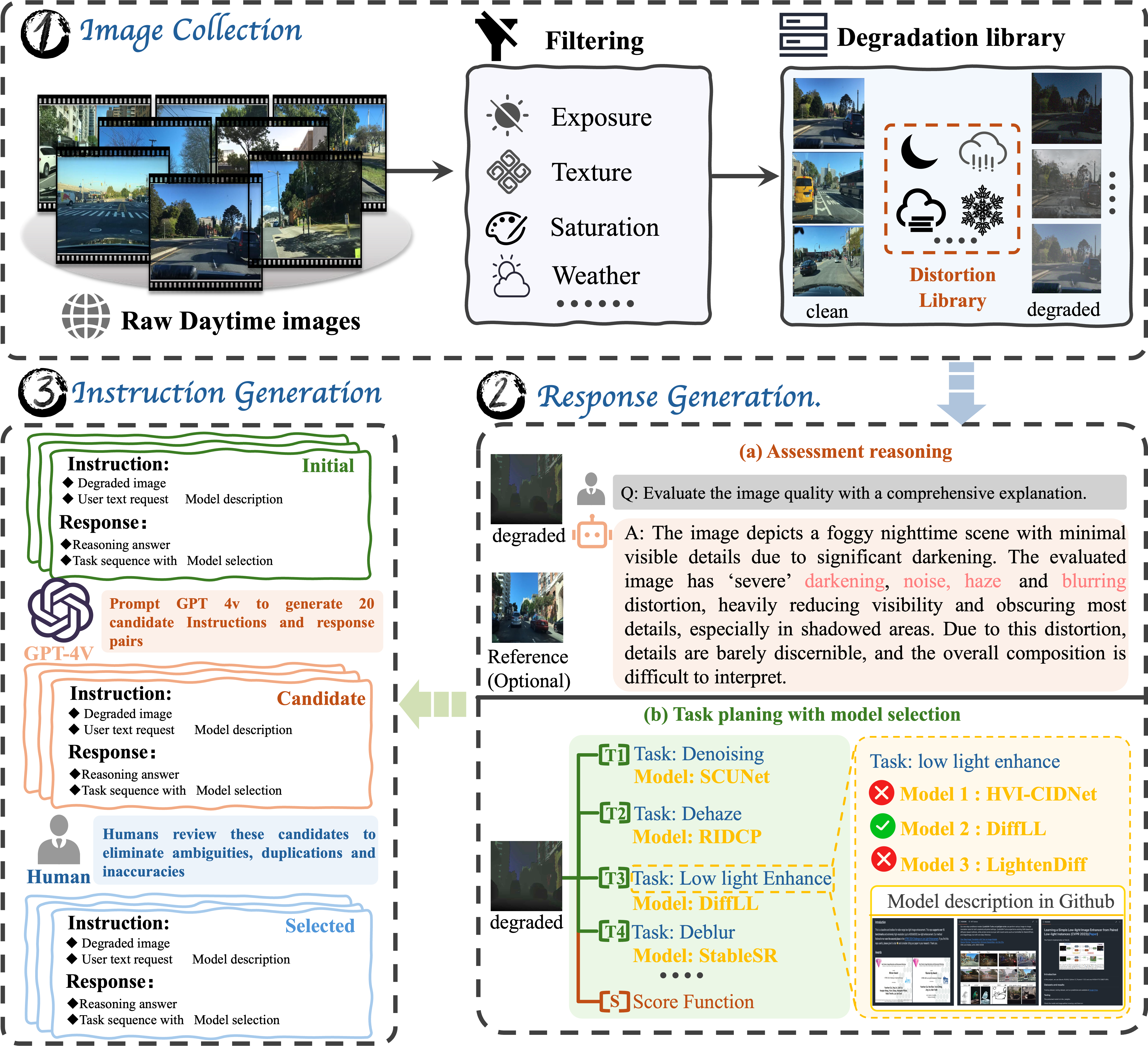
Figure 3: The dataset construction workflow consists of three main steps: 1) Synthesis of degraded images. 2) Generation of Assessment reasoning and the optimal task sequence. 3) Generation of instruction-response pairs for the system.
Paper
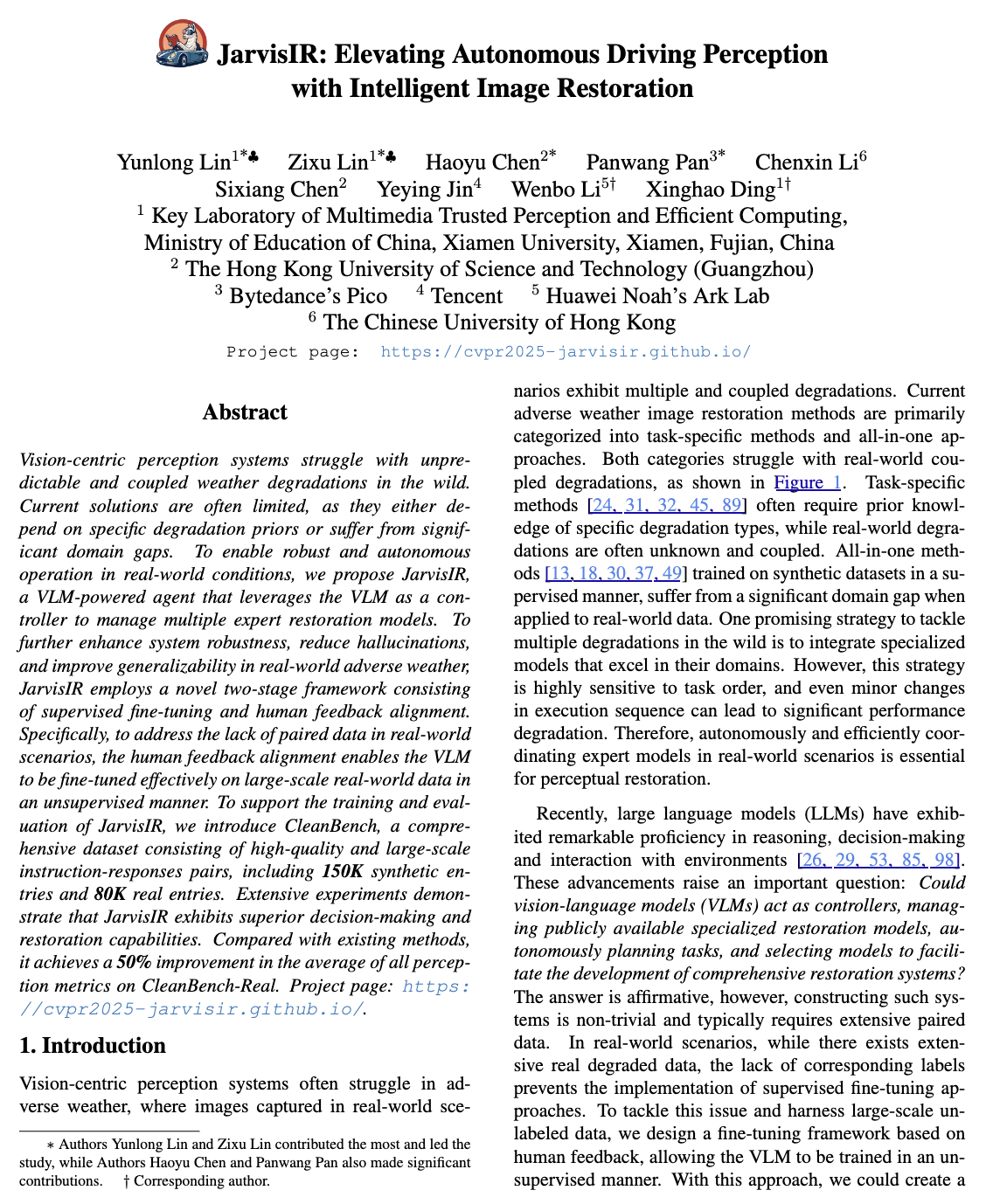